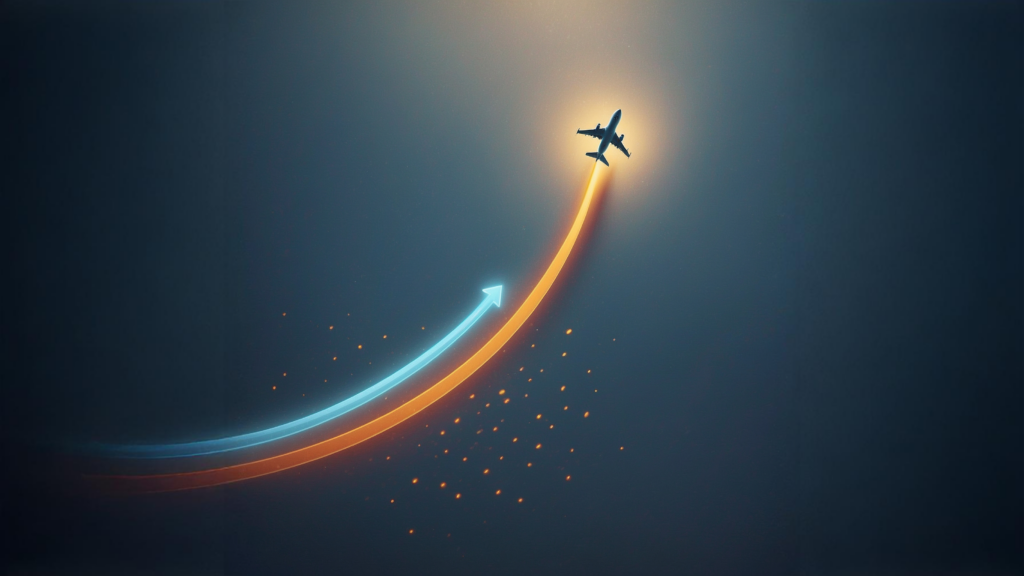
When I started my role as a Project Engineer at Bombardier, I didn’t expect it to be the first step in my journey toward becoming a data scientist. At the time, my focus was on traditional engineering tasks—managing processes, collaborating with cross-functional teams, and ensuring that the Global 7500 project stayed on schedule. But as challenges arose, I found myself drawn to data-driven problem-solving, and that curiosity gradually transformed my career.
One of my primary responsibilities was handling RFCs—Request for Change reports. These documents tracked modifications to aircraft designs and processes, often running into the hundreds. Initially, the process of generating these reports was manual, prone to errors, and time-consuming. I remember the frustration of pulling data from multiple sources, cross-referencing spreadsheets, and formatting the final summaries. Stakeholders needed timely, accurate insights, but the system we had wasn’t cutting it.
Driven by the need to improve this process, I decided to dive into SQL. I taught myself the basics and started experimenting with queries to pull data directly from our central database. My first breakthrough was automating the Weekly RFC Status Summary. What used to take hours now ran in minutes, delivering consistent results with zero errors. It wasn’t just about saving time—it was about ensuring accuracy, which, in an industry as precision-driven as aerospace, was non-negotiable.
With every success, I got bolder. Soon, I was developing more complex reports, like the Top Modification Summary, which ranked high-impact changes by cost, complexity, and critical path influence. The insights from these automated reports began shaping key decisions. For the first time, my work wasn’t just about managing processes—it was influencing the strategic direction of the project. This was my “aha” moment: data wasn’t just a tool; it was a catalyst for better decisions.
As these reports became more integral, my exposure to upper management increased. I was invited to meetings with senior stakeholders, where my insights were not only valued but actively sought after. Discussions about RFC trends, cost impacts, and approval efficiencies became conversations where I contributed not as an observer, but as a driver of strategy. It was exhilarating—and a little intimidating—to see how my work impacted the broader vision of the company.
One of the most memorable moments came when I developed the Cost Impact Reporting System for RFCs. By linking technical data with financial projections, I was able to forecast cost overruns with a level of precision that hadn’t been possible before. This report flagged a set of pending changes that, if implemented together, would have exceeded the budget. Thanks to those insights, the project team re-prioritized, saving the company substantial costs. I realized then that this wasn’t just about technical expertise—it was about creating value at the organizational level.
The impact of my work didn’t stop there. Automating daily RFC snapshots allowed teams to get real-time updates, improving cross-functional communication and reducing delays. Errors dropped significantly because manual intervention was minimized. Teams trusted the data more, and I found myself drawn further into the world of data analytics. I wanted to know more: how could I uncover hidden patterns, predict outcomes, and make systems even smarter?
This curiosity led me to explore predictive analytics and machine learning. I started learning Python on the side, experimenting with models to identify trends in RFC approvals. It wasn’t long before I realized that data science wasn’t just a skillset—it was a way of thinking, a way to approach problems that combined creativity with technical rigor.
By the time I left Bombardier, I was no longer just a Project Engineer—I was a data-driven strategist. My journey into data science wasn’t a sudden leap but a series of incremental steps, each driven by a desire to solve real-world problems. Today, when I analyze datasets, build models, or develop dashboards, I carry with me the lessons I learned during those early days: that data, when harnessed effectively, isn’t just a tool for efficiency—it’s a key to transformation.